12 SES class findings
brief overview of student findings exploring the corpus
12.1 class findings
Student | Child.Code | Age | Prepositions | Articles | Conjunctions | Paraphrase.with.verb | Hesitation.phenomena..Pauses..repeated.articles |
---|---|---|---|---|---|---|---|
Griechische Kinder | NA | NA | NA | NA | NA | NA | NA |
Laura | GCC | 9 | auf: 3, an: 1, in: 7, nach: 1, zu: 7 - zun: 1, hinter:0, neben:0, vor:1 | NA | NA | NA | Viele Pausen, häufiges Zögern |
NA | GDC | 8 | auf: 12, an:1, in 19 anstatt im:1, nach:5, zu:1, hinter:0, neben:0, vor:1 | NA | NA | NA | viele Pausen |
NA | GCG | 9 | NA | NA | NA | NA | überlegt oft kurz, wenn sie nicht genau weiß, was sie zunächst sagen wird |
NA | GDD | 9 | NA | NA | NA | NA | NA |
Türkische Kinder | NA | NA | NA | NA | NA | NA | NA |
Laura | TAC | 12 | auf: 16, an: 2, in: 6, nach: 4, zu: 7, hinter: 0, neben: 2, vor: 1 | NA | NA | NA | wenig Pausen oder Zögern |
NA | TBF | 12 | NA | NA | NA | NA | NA |
NA | TAI | 13 | auf: 8, an: 1, in: 12, nach: 5, zu: 3, hinter: 1, neben: 3, vor: 1 | NA | NA | NA | Viele Pausen, häufiges Zögern |
NA | TBB | 14 | NA | NA | NA | NA | NA |
12.2 class findings
Student | Child.Code | Age | Prepositions | Noticing | Self.correction..content.or.form. | Interviewer | More.information |
---|---|---|---|---|---|---|---|
Griechische Kinder | NA | NA | NA | NA | NA | NA | NA |
Katharina | GDA | 11 | NA | 0016 bis 0022 (answers INT not transcripted)//GDA: Hier, das Maedchen und ein Jung basteln einen Schneemann. Das Maedchen macht ein Mohrruebe fuer Nase. Und hier gibt sie…@ ’ne…@ ’ne Stock 90 obj i.o.. //INT: was ist das? //GDA: Das hier? hm //INT: was macht man damit? //GDA: Machen sauber. //INT: Genau, n Besen./[später:] und nicht den Besen/–>Bedeutungsverhandlung: gemeinsam Semantik umschreiben und erfassen; dann Vorschlag, der aufgegriffen wird/ | 0194 [Drachen] wenn es ganz gut Luft ist, Luft gib’s, dann… /0237 Hier fragt ein Frau…@ den Schna- Schaffner wo geht da, der Zug | lacht viel, macht Späße, fragt freundlich nach, stimmt, genau/Recasts/0096 (answer not transcripted) Er faellt sich um. Hmh, fällt runter. (Übergeneralisierung reflexive Verben)/0104 (answer not transcripted) [der Junge] fangt. Ja, fängt. /0181 (answer not transcripted) Soll ich sagen, wohin sie gehört? Ja, dann ist da noch irgendwas, was dazu gehört./0182 (answer not transcripted) Hier sind so Kristall. Eis. Ja genau, Eis. Eiskristalle | NA |
Türkische Kinder | NA | NA | NA | NA | NA | NA | NA |
Katharina | TAF | 13 | NA | Zeile 0059 (Chat file) Kopf/–> Noticing, Pause + direkter Vorschlag – lernt direkt etwas neues/0091 (Chat) Besen/–> Vorschlag, der direkt übernommen wird./0103 0104 (Chat) Mohrrübe/ | Sie setzt sich im einen Wagen und der Maedchen zieht sie- zieht er. (0068)/Ja, wenn du kein Fahrer- Fahrkarte hast, dann musst du wieder aussteigen. (0163)/weil, er traegt viel schwerer und er traegt (…) langs- ne bisschen leichter (0131) /da finden die- da find der Junge sein Vater und Mutter (0179)/–> Umstrukturierung Syntax auch für L1 typisch | Interviewer sagt v.a. ok, gut, hmh, prima, alles ist erlaubt, lacht, ermutigt bei langen Pausen, weiterzumachen//Nachfrage Zeile 0038 (Chat) Irrenhaus//Recast 0146 (answer from INT not transcipted)//der Apfel gehoert die Aepfeln. Und warum gehören die beiden zusammen? | Viele und lange, ungefüllte Pausen |
NA | TBV | 14 | NA | NA | NA | NA | NA |
NA | TBE | 13 | NA | NA | NA | NA | NA |
NA | TBF | 12 | NA | NA | NA | NA | NA |
NA | TBM | 13 | NA | NA | NA | NA | NA |
NA | TBN | 14 | NA | NA | NA | NA | NA |
12.3 class findings
Student | Child.Code | Age | Prepositions | Articles | Conjunctions | Hesitation.phenomena..Pauses..repeated.articles | Self.correction..content.or.form. |
---|---|---|---|---|---|---|---|
Griechische Kinder | NA | NA | NA | NA | NA | NA | NA |
Miriam | GCA | 8 | auf: 3/an: 1 -> am: 1/in: 31/nach: 4/zu: 3/hinter: 1/neben:/vor: 0 | Reduced forms ///n: 1 -> ein/einen | und: 95/dann: 11/danach: 0/weil: 1 | Viele Pausen, häufiges Zögern | Wenig Selbstkorrekturen |
NA | GCE | 11 | auf: 0/an: 0/in: 0/nach: 0/zu: 0/hinter: 0/neben: 0/vor: 0 | Reduced forms /// | und: 180/dann: 25/danach: 2/weil: 3 | Wenig Pausen oder Zögern | Mehr Selbstkorrekturen |
NA | GDE | 10 | auf: 31/an: 0/in: 49 -> inzu: 1/nach: 5/zu: 26 -> inzu: 1/hinter: 3/neben: 2/vor: 3 | Reduced forms ///n: 4 -> ein/einen//ne: 5 -> eine//s | und: 57/dann: 13/danach: 1/weil: 9 | Seit 10 Jahren in Deutschland/Keine Audio-Datei | NA |
NA | GDF | 11 | auf: 10/an: 0/in: 25/nach: 6/zu: 15/hinter: 2/neben: 2/vor: 2 | Reduced forms / | Und/Und dann/danach/weil | Wenig Pausen oder Zögern | Bewusste Selbstkorrekturen |
Türkische Kinder | NA | NA | NA | NA | NA | NA | NA |
Miriam | TAA | 13 | auf: 16/an: 2/in: 6/nach: 4/zu: 7/hinter: 0/neben: 2/vor: 1 | Reduced forms / | Und/Und dann/danach/weil | Wenig Pausen oder Zögern | Inhaltliche Selbstkorrekturen, weniger grammatikalisch |
NA | TAD | 14 | auf: 2 -> aufm: 1/an: 0/in: 5 -> inne: 1/nach: 2/zu: 6/hinter: 1/neben: 2/vor: 0 | Reduced forms / | Und/Und dann/danach/weil | Viele Pausen, häufiges Zögern | Wenig Selbstkorrekturen |
NA | TBC | 14 | auf: 14/an: 6/in: 9/nach: 2/zu: 16/hinter: 3 -> hinterher: 1/neben: 4/vor: 0 | Reduced forms / | Und/Und dann/danach/weil | Viele Pausen, häufiges Zögern | Wenig Selbstkorrekturen |
NA | TBD | 13 | auf: 14/an: 0/in: 18/nach: 6/zu: 7/hinter: 0/neben: 1 -> daneben: 1/vor: 2 | Reduced forms / | Und/Und dann/danach/weil | Wenig Pausen oder Zögern | Wenig Selbstkorrekturen |
Carol | test-ok | NA | NA | NA | NA | NA | NA |
12.4 principle of accountability 2
roughly: the number of occurences of one (coded nonstandard) feature over the number of total instances of the feature (including standard + nonstandard realisations). e.g.:
token | instances | standard | nonstandard | normalised |
---|---|---|---|---|
schnee | 54 | 33 | 21 | 38.8888889 |
all | coded “FALSCH” | coded “1” (feature = TRUE) | percent (D2/B2\\\*100) |
workflow:
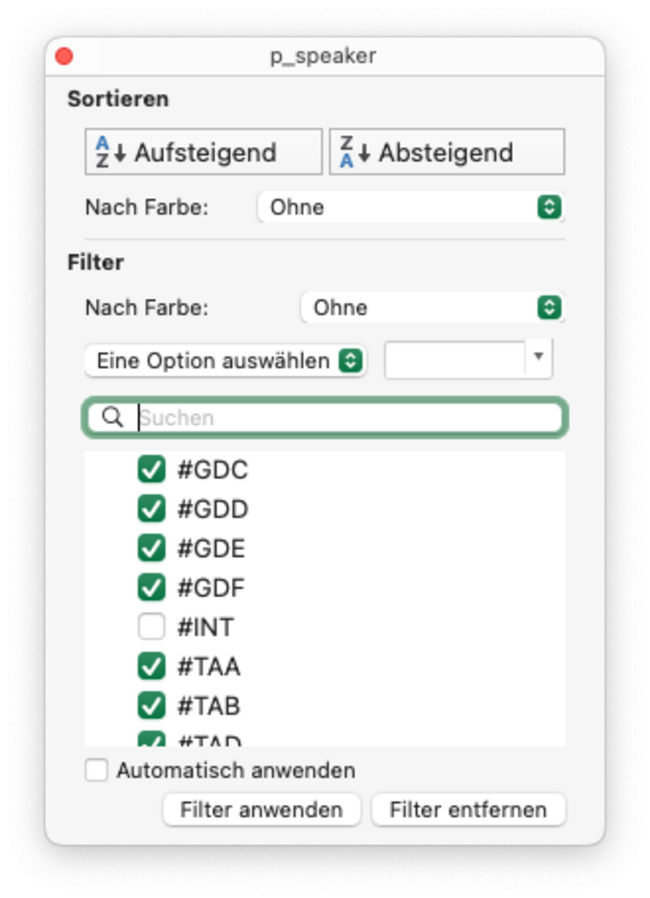
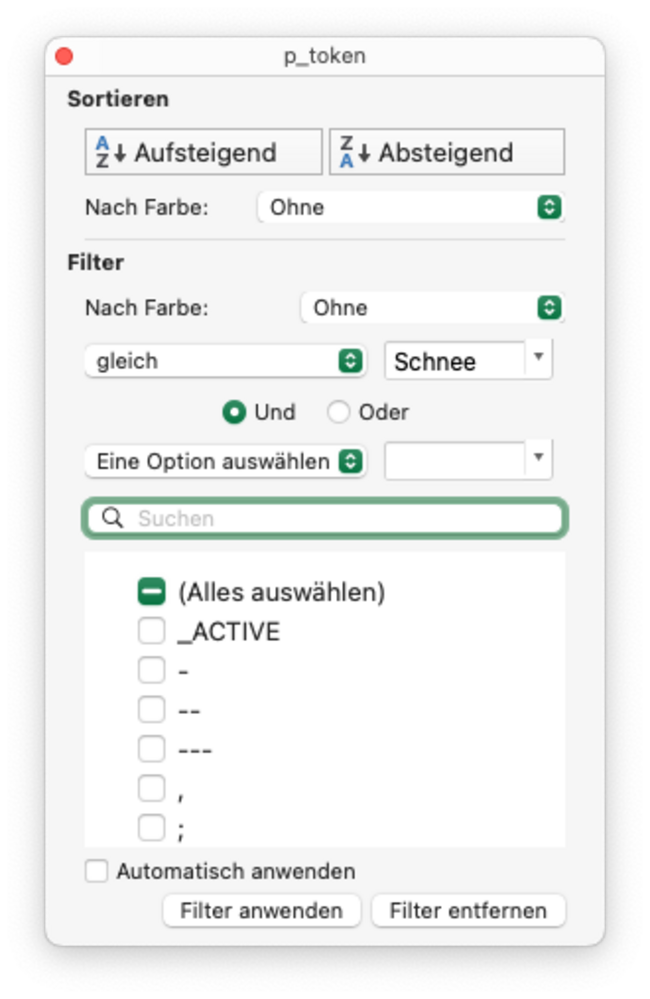
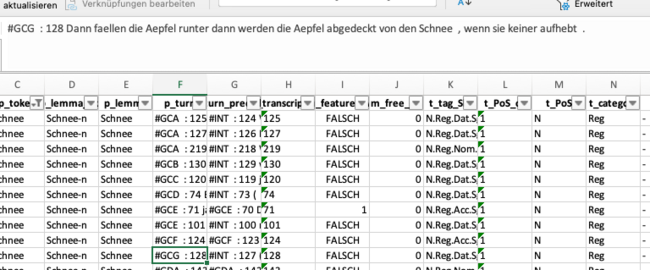
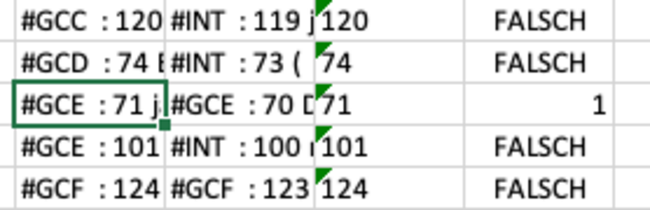
- filter out #INT speaker
- filter after token [schnee]
- analyse turn and define wether standard or nonstandard feature
- set evaluation column to 1=TRUE for nonstandard occurences
- sum up number of total instances
- sum up number of positives (nonstandard realisation)
- compute number of negatives (standard)
- compute percentage
12.5 SES distribution analysis
the following is the output table of the multivariate analysis of a frequency table of all feature codes over all target childs.
the frequency table was exported from an ANNIS installation of the SES corpus. the query for getting the proper results is:
codetag = /c_.*/ & int = /T.*|G.*/ & #1 . #2
this outputs all occuring codes over the transcripts and associates them to the speaker, either T=any turkish or G=any greek. with that you get a frequency table looking like this (exerpt):
featurecode | child | count |
---|---|---|
NPR | GCA | 16 |
COM | TBR | 16 |
COM | TAA | 15 |
0AR | TBL | 14 |
NPR | TBT | 14 |
0AR | GCB | 14 |
COM | TBL | 14 |
0AR | GDA | 13 |
COM | TBM | 13 |
NNS | TBQ | 13 |
0AR | TBS | 13 |
COM | GCA | 13 |
NPR | TBU | 12 |
NPR | GCC | 12 |
0AR | TBT | 12 |
COM | TBU | 12 |
0AR | TBU | 11 |
NNS | GCB | 11 |
visualised:
12.5.1 small significance testing:
script source: https://github.com/esteeschwarz/HU-LX/blob/main/scripts/distribution-analysis.R
the applied lmer (linear mixed effects regression model) 3 formula is:
count ~ feature + (1 | L1)
in words: we posited a main effect of feature and random effects of L1.
with this assumption a significance of p < 0.05
was tested at 0AR (zero article), i.e. the coding of this feature (here) depends significant on the L1 use of the target child. IMPORTANT: this does not allow general statements about the relation of 0AR feature and L1 since the transcript corpus was not coded/annotated until every instance of each feature.
12.5.2 lmer coefficients
Estimate | Std. Error | df | t value | Pr (>t) | |||
feature0AR | 5,324 | 2,778 | 333 | 1,916 | 0,056 | ||
featureNPR | 4,286 | 2,786 | 333 | 1,538 | 0,125 | ||
featureNAG | 3,353 | 2,817 | 333 | 1,19 | 0,235 | ||
featureNNS | 2,8 | 2,792 | 333 | 1,003 | 0,317 | ||
feature0SU | 2,571 | 2,777 | 333 | 0,926 | 0,355 | ||
featureNGN | 1,893 | 2,786 | 333 | 0,679 | 0,497 | ||
featureNPE | 2 | 2,957 | 333 | 0,676 | 0,499 | ||
feature0PR | 1,682 | 2,799 | 333 | 0,601 | 0,548 | ||
feature0OB | 1,261 | 2,797 | 333 | 0,451 | 0,652 | ||
featureNSM | 1,167 | 2,794 | 333 | 0,418 | 0,677 | ||
featureNWO | 1,067 | 2,828 | 333 | 0,377 | 0,706 | ||
(Intercept) | 1 | 2,738 | 333 | 0,365 | 0,715 | ||
feature0EX | 1 | 3,353 | 333 | 0,298 | 0,766 | ||
feature0MD | 1 | 3,872 | 333 | 0,258 | 0,796 | ||
featureNPO | 1 | 3,872 | 333 | 0,258 | 0,796 | ||
featureNVP | 0,667 | 2,957 | 333 | 0,225 | 0,822 | ||
featureNEX | 0,6 | 2,999 | 333 | 0,2 | 0,842 | ||
feature0RF | 0,5 | 3,353 | 333 | 0,149 | 0,882 | ||
featureNCM | 0,429 | 2,927 | 333 | 0,146 | 0,884 | ||
feature0CP | 0,375 | 2,822 | 333 | 0,133 | 0,894 | ||
feature0AU | 0,333 | 2,957 | 333 | 0,113 | 0,91 | ||
feature0VP | 0,308 | 2,841 | 333 | 0,108 | 0,914 | ||
featureNRL | 0,267 | 2,828 | 333 | 0,094 | 0,925 | ||
featureNPV | 0,091 | 2,86 | 333 | 0,032 | 0,975 | ||
feature0AP | 0 | 3,872 | 333 | 0 | 1 | ||
feature0EL | 0 | 3,872 | 333 | 0 | 1 | ||
feature0PD | 0 | 3,872 | 333 | 0 | 1 | ||
feature0PN | 0 | 3,061 | 333 | 0 | 1 | ||
feature0PT | 0 | 3,872 | 333 | 0 | 1 | ||
feature0RL | 0 | 3,872 | 333 | 0 | 1 | ||
feature0RP | 0 | 3,872 | 333 | 0 | 1 | ||
featureNAR | 0 | 2,927 | 333 | 0 | 1 | ||
featureNAU | 0 | 3,872 | 333 | 0 | 1 | ||
featureNCA | 0 | 3,872 | 333 | 0 | 1 | ||
featureNCJ | 0 | 3,872 | 333 | 0 | 1 | ||
featureNCN | 0 | 3,872 | 333 | 0 | 1 | ||
featureNCP | 0 | 3,872 | 333 | 0 | 1 | ||
featureNEA | 0 | 3,872 | 333 | 0 | 1 | ||
featureNIO | 0 | 3,872 | 333 | 0 | 1 | ||
featureNMD | 0 | 3,872 | 333 | 0 | 1 | ||
featureNNN | 0 | 3,872 | 333 | 0 | 1 | ||
featureNPN | 0 | 3,872 | 333 | 0 | 1 | ||
featureNPS | 0 | 2,957 | 333 | 0 | 1 | ||
featureNVC | 0 | 3,872 | 333 | 0 | 1 |
Bates u. a., „Fitting Linear Mixed-Effects Models Using lme4“. 2015. doi: 10.18637/jss.v067.i01↩︎
Bates u. a., „Fitting Linear Mixed-Effects Models Using lme4“. 2015. doi: 10.18637/jss.v067.i01↩︎